March 21, 2018
Crop growth models, statistical learning and big data to be featured in Elmer G. Heyne Crop Science Lecture March 27
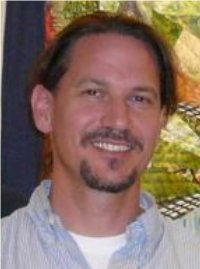
Charlie Messina, internationally noted senior research scientist with DuPont Pioneer, will present the 34th Elmer G. Heyne Crop Science Lecture at 4 p.m. Tuesday, March 27, in 1018 Throckmorton Hall.
Messina will present "On the Fusion of Crop Growth Models and Statistical Learning: Leveraging Plant Science to Enhance Genome‐to‐Phenome Prediction in the Era of Big Data in Agriculture." Refreshments will be served at 3:30 p.m. in the first floor lobby of Throckmorton Hall. The lecture is open to the public.
Messina has a doctorate in agriculture and biological engineering from the University of Florida, and master's and bachelor's degrees from the University of Buenos Aires. His major research interests include the fusion of biological models and statistical learning methodologies to increase the limits of predictability in dynamic agricultural systems — e.g. crop growth model and whole genome prediction methodology; physiology and genetics in maize; and development and application of mathematical models for prediction and analyses of agricultural systems response to environmental change.
Messina will discuss how plant science underpins technological developments that are instrumental for the improvement of life for the world's poor and society in general. Current rates of genetic gain and agricultural intensification are not enough to produce food, fiber and fuel to satisfy the projected demands. Recent advancements in information technologies, phenomics, and statistical learning algorithms make large datasets available for analysis and motivate prediction and classification studies in agriculture.
Messina will further discuss a formal generalized model that enables the integration of genomic, phenomic, environmental data and biological knowledge to predict the performance of maize hybrids. The model has core elements of crop modeling and statistical learning in the form of a hierarchical Bayesian algorithm. The model is demonstrated with case studies in breeding and biotechnology for improved drought tolerance. Forecasts of the consequences of variation in the genetic makeup of maize is conducted at the regional scale to demonstrate the methodology.